Artificial intelligence (AI) and machine learning (ML) technologies are revolutionizing many fields, and cardiovascular medicine is no exception. A major focus across academia, industry, and government agencies is applying these advanced analytical tools to enable more precise approaches to treating heart disease.
The American Heart Association has released a comprehensive scientific statement outlining the current state-of-the-art and future potential for using AI/ML in cardiology care. Let’s dive into some key areas where AI could have a transformative impact.
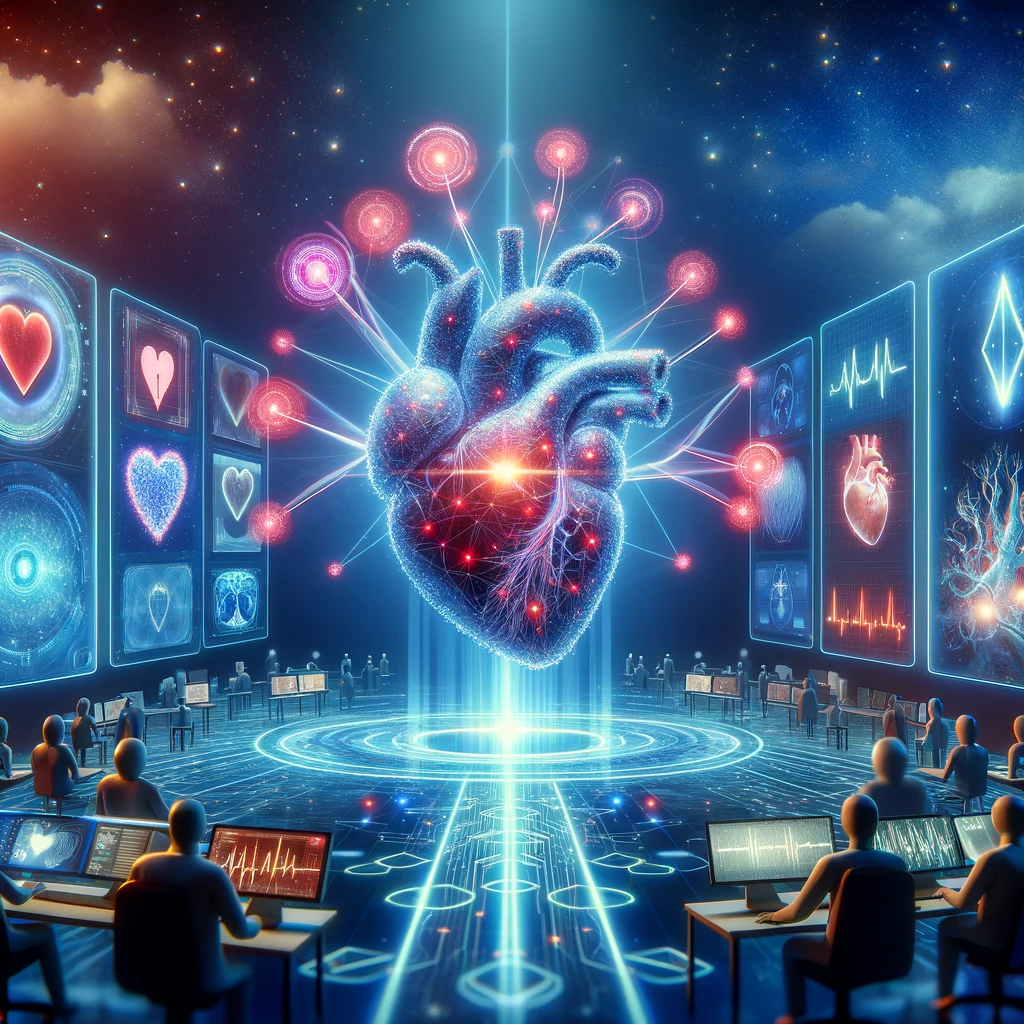
AI in Cardiac Imaging
Imaging with echocardiography, CT, MRI, and nuclear medicine are essential diagnostic tools. However, highly trained experts must acquire, process, and interpret the data. AI/ML algorithms can help automate many of these labor-intensive steps, from segmenting cardiac structures to quantifying function to detecting disease. Emerging applications include:
- Automated calculation of parameters like ejection fraction and strain
- Identifying wall motion abnormalities suggestive of prior heart attacks
- Differentiating hypertrophic cardiomyopathy from physiologic hypertrophy
- Quantifying coronary plaque burden and blood flow
- Predicting sudden cardiac death risk from patterns of fibrosis on MRI
- Planning electrophysiology procedures by mapping structural disease
By automating routine tasks, AI has the potential to increase throughput, reduce radiation exposure, and broaden access to high-quality cardiac imaging. This can improve access to care especially in underserved areas lacking specialized expertise.
However, challenges remain in curating large, high-quality, diverse imaging datasets to train AI models that will generalize well to new patients. Robust validation across different population groups is also critical before widespread clinical deployment.
Electrocardiogram (ECG) Analysis
The standard 12-lead electrocardiogram is ubiquitous in cardiology, yet interpreting ECGs is increasingly difficult given the scale of data and limited human expertise. AI/ML algorithms show immense promise in this domain, both for automating routine ECG readings and detecting subtle patterns that may reveal early signs of disease. This is another exciting area for Artificial intelligence in cardiology.
AI/ML algorithms have demonstrated the ability to identify cardiac pathologies without direct imaging. They can identify left ventricular dysfunction, hypertrophic cardiomyopathy, cardiac amyloidosis, and pulmonary hypertension from the raw ECG signal alone. Sometimes, signs of pathology can appear on the ECG years before those diagnoses become clinically apparent. Other algorithms can predict atrial fibrillation risk and stroke likelihood in patients still in normal sinus rhythm.
Beyond disease detection, AI/ML techniques applied to the ECG enable new phenotyping methods, such as inferring a patient’s biological age and correlating that with health factors like ejection fraction and coronary disease. Such findings could potentially guide preventive interventions.
As with imaging, a major hurdle is validating AI/ML ECG algorithms across diverse populations to ensure they perform consistently regardless of factors like age, sex, race, and ethnicity. Developing robust human-AI interfaces to explain AI decision-making will also be essential for building clinician trust and adoption.
Physiologic Monitoring
Hospitalized patients are continuously monitored with devices tracking vital signs like heart rate, respiratory rate, and oxygen levels. Traditional monitoring systems rely on simple threshold-based alarms, an approach with major limitations.
Applying AI/ML techniques to these multi-parameter physiologic data streams allows extracting higher-order signatures and relationships between variables that may indicate a patient is on a trajectory toward deterioration. AI/ML algorithms can stratify patients into high-risk phenotypes. It can also detect physiologic changes earlier than simple threshold alerts, potentially predicting adverse events like cardiac arrest hours in advance.
While still an emerging field, AI/ML monitoring approaches could fundamentally alter how hospitals triage resources and escalate care. Major challenges involve:
- Validating monitoring algorithms across diverse health systems.
- Ensuring they don’t perpetuate demographic biases.
- Integrating AI tools into established clinical workflows.
Other Opportunities for AI in Cardiology
The statement also highlights additional opportunities for applying AI/ML in areas like managing medical documentation through natural language processing of reports, designing adaptive clinical trials, and better phenotyping disease to enable precision medicine.
Importantly, the authors emphasize that as these technologies advance, principles of ethical AI, data privacy, algorithmic fairness, and user education must keep pace to ensure equitable and appropriate deployment of AI in cardiology.
Conclusion
The American Heart Association’s statement provides a comprehensive overview of how AI and machine learning could transform multiple facets of cardiovascular care. While still an emerging field with significant challenges to overcome, these techniques have immense potential to augment clinical expertise, improve diagnostic capabilities, optimize treatment pathways, and ultimately enhance patient outcomes. Continued research, validation, and collaborative efforts across stakeholders will be crucial to realizing this potential over the coming years.
Check out our other articles!
Ai in Primary Care with Google’s AMIE: The PCP of the Future
AI Solving Medical Error: Why AI is Important in Healthcare
Harnessing AI: Large Language Models Transforming Healthcare Delivery
Can AI Save Science? Healing the Wounds of Academic Publishing